How On-Board Data Processing is Reshaping Space Missions
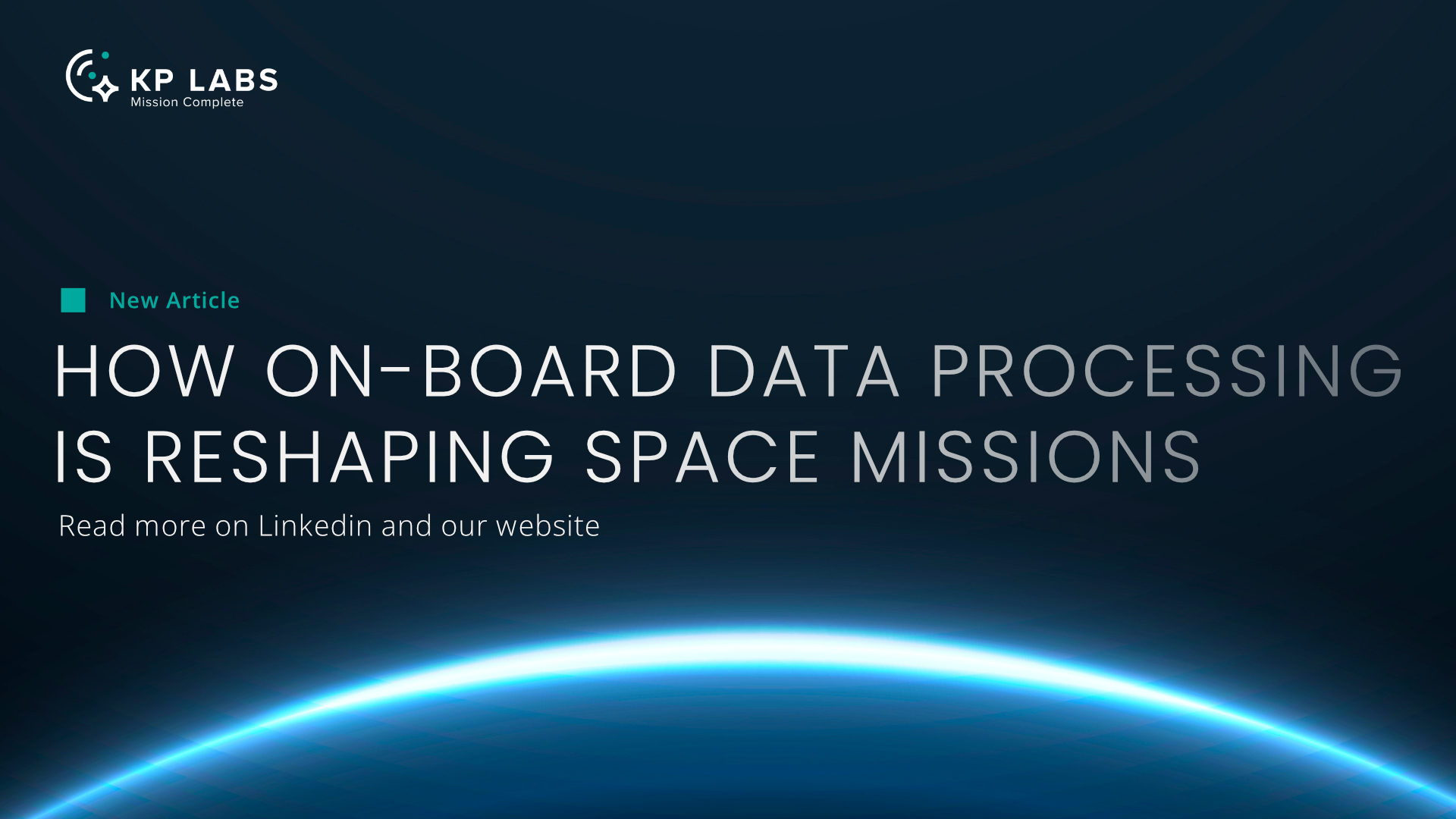
The space sector is witnessing a paradigm shift. With an exponential increase in the volume of data collected by satellites, we are approaching a bottleneck — not because we lack sensors, but because the traditional data transmission model still limits us. The prevailing approach has long been to downlink raw data from space to Earth and process it afterwards. But this model is straining under the pressure of higher-resolution sensors, longer mission durations, and a growing need for near-real-time insights. Enter on-board data processing: a technology and a mindset that changes how we think about data in orbit, offering a fundamental redefinition of mission efficiency, autonomy, and scientific value.
On-board data processing is not only about artificial intelligence, although AI certainly plays an enabling role. It's about proximity — bringing the "brain" closer to the "eyes" of space systems. Processing data directly on the satellite, even at a preliminary level, provides a decisive advantage: time. When satellites are equipped with the capability to process or preprocess the information they collect, they can transmit only the most relevant results or intermediate products to the ground. This shift not only reduces downlink costs and energy usage but also increases the responsiveness of space systems. In missions where time is critical — for example, in disaster monitoring or planetary exploration — the latency introduced by traditional pipelines can render valuable data obsolete by the time it reaches Earth [3].

This need is particularly evident in Earth observation (EO) missions, where hyperspectral imaging data can reach sizes of several gigabytes per acquisition. In our studies, we showed that such data's efficient transfer and storage is costly and slow. It has been demonstrated that only a fraction of the spectral bands typically contains actionable information, and therefore, sending full raw hyperspectral cubes to Earth is wasteful. On-board algorithms — such as cloud detection, band selection, or segmentation — can drastically reduce transmitted data volume without compromising utility.
The benefits of on-board processing become even more apparent when considering mission scalability. Constellations of satellites or collaborative architectures such as scout-mothership systems can share context in real time, exchange processed information, and dynamically adjust their operations. In recent ESA-funded research led by KP Labs in collaboration with IBM Research Europe, a concept was explored where a low-resolution "scout" nanosatellite (with 100 m/pixel resolution and a wide field of view) continuously scans the Earth's surface and identifies regions of interest. These areas are then transmitted to a higher-resolution "mothership" microsatellite (with 1–3 m/pixel resolution) that can adjust its viewing angle and spectral settings to acquire targeted, high-fidelity observations. This division of labor, enhanced by inter-satellite communication and edge analytics, enables smart tasking and rapid response to dynamic events like wildfires or floods [1].
At KP Labs, we have explored and implemented on-board data processing through several ongoing and planned missions. Intuition-1, our 6U hyperspectral nanosatellite launched in 2023, was designed to test the potential of deep learning models executed directly in orbit. Equipped with the Leopard data processing unit, the satellite can analyze hyperspectral data before transmission. This results in a significantly reduced data load, improved responsiveness, and the ability to perform tasks like anomaly detection or cloud filtering in near real-time. The mission demonstrated the on-board execution of machine learning models for determining agricultural features and classifying land cover types (such as bare soil), showcasing how AI can transform preprocessed data into valuable insights mid-flight. To enable such AI inference, raw hyperspectral images — particularly from push-broom sensors — must first undergo rigorous preprocessing steps. These include radiometric correction, geometric co-registration, noise filtering, and construction of a continuous image cube from narrow swaths acquired line-by-line. Only with such cleaned and normalized data can deep learning models operate reliably on board [3], and only when benchmarked thoroughly [4].

Its primary on-board use case was focused on soil parameter estimation using hyperspectral data, developed as part of the HYPERVIEW Challenge — a competition organized by KP Labs, ESA, and partners, which attracted over 150 global teams [5]. Intuition-1 supports CCSDS-123 compression standards — a conventional but still effective technique for reducing hyperspectral data volume — complementing more advanced AI-based methods to minimize transmission load. It also features mission-adaptive on-board learning, allowing in-orbit model updates based on changing environmental conditions.
Another illustrative example is the ESA’s Phi-Sat-2 mission, which employed a neural network based on the U-Net architecture to discard unusable data — in this case, images obscured by clouds — before they ever reached the ground segment. This relatively straightforward application of AI brought tangible benefits: reduced bandwidth usage, faster data availability, and less storage overhead on the ground. The elegance of this approach lies in its effectiveness: not every on-board model has to be complex in logic to deliver impactful results. In this case, deep learning-based cloud masking, rather than classical filtering, was sufficient to dramatically increase mission efficiency [6].
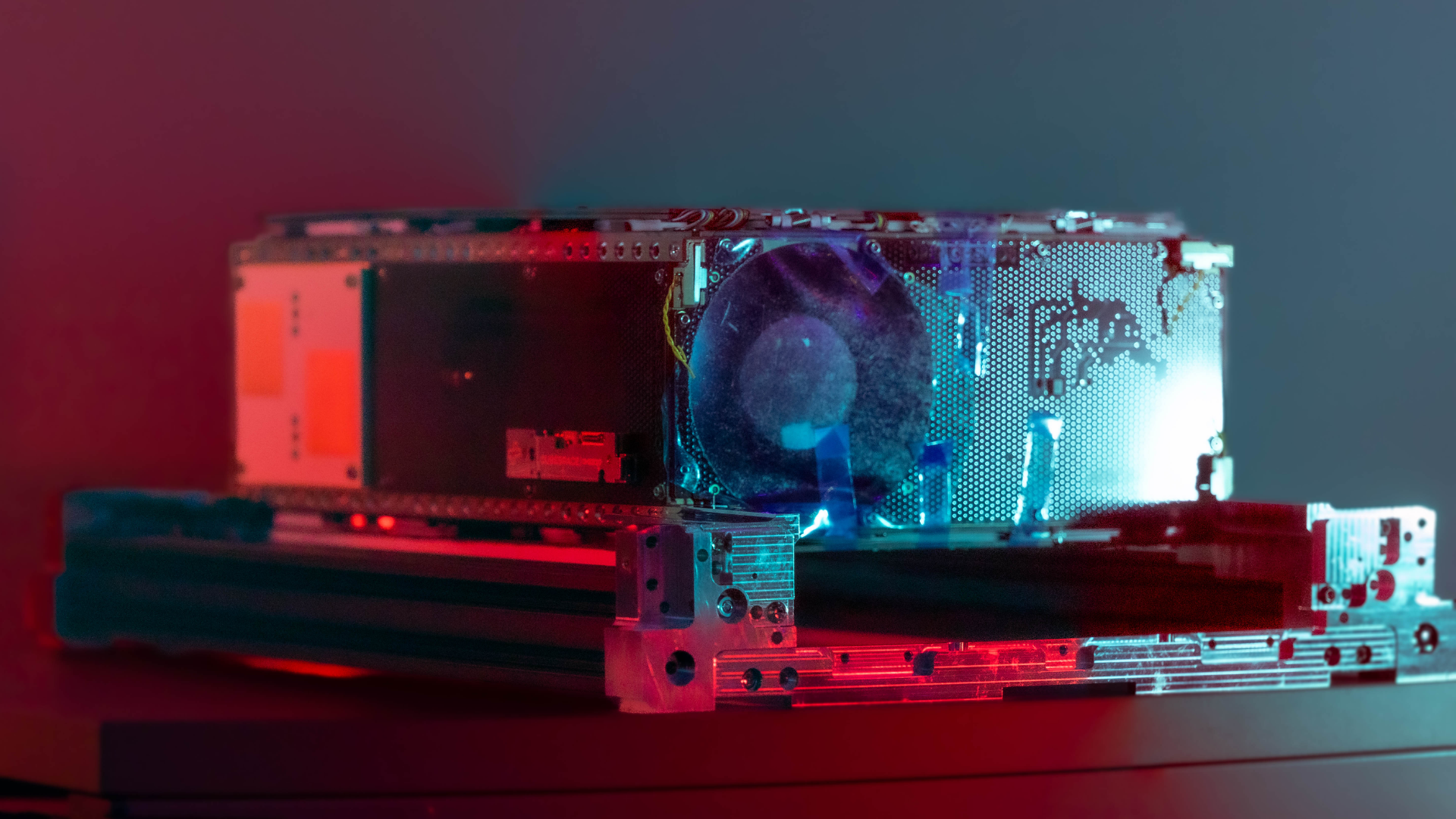
On-board processing also plays a critical role in deep-space exploration, where the distance from Earth introduces significant communication delays. In the M-ARGO mission — a European Space Agency study in which KP Labs is a participant — an autonomous cubesat will be deployed to study an asteroid. Given the communication constraints, the spacecraft must be able to make decisions locally, including navigation and scientific data prioritization. These constraints make on-board data handling essential, as decisions about what data to transmit — and when — can mean the difference between a successful flyby and a missed opportunity. In this context, edge computing is not a luxury but a mission-enabling requirement.
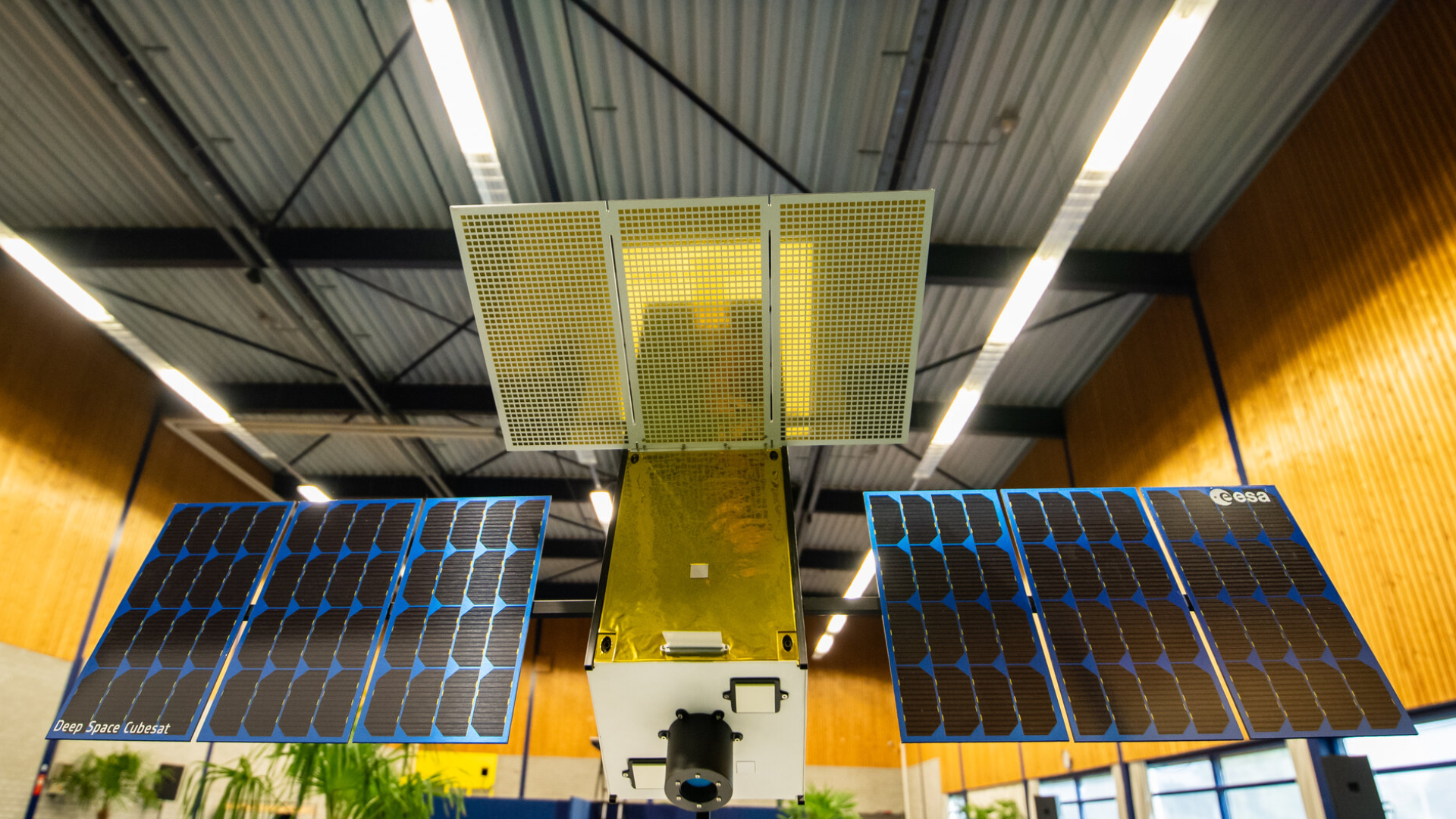
LeopardISS, KP Labs' latest contribution to the on-board processing revolution, continues this trajectory by bringing AI experimentation directly to the International Space Station. As part of the IGNIS mission — Poland's first scientific mission to the ISS — LeopardISS enables the validation of data processing and AI models in low Earth orbit under long-duration, real operational conditions. Housed within the European Columbus module's ICE Cubes Facility, LeopardISS is powered and networked continuously, allowing for remote updates, real-time testing, and iterative development. Its architecture is based on KP Labs' Leopard platform, refined for compact, modular deployment in constrained environments like the ISS. The system includes a Xilinx chipset with FPGA acceleration supported by Vitis AI and can execute neural network inference with up to 0.3 TOPS/W energy efficiency. Like Intuition-1, LeopardISS supports CCSDS-123 compression but enables real-time classification and segmentation directly on board. Beyond internal R&D, LeopardISS also hosts research from the Poznań University of Technology, where scientists are developing 3D image analysis techniques for planetary rovers — a critical step toward self-navigating surface explorers. With Polish astronaut Sławosz Uznański-Wiśniewski scheduled to oversee the platform during the mission, the experiment validates hardware. It opens doors for future integration of AI-capable systems on planetary and orbital missions.

This shift toward autonomy and distributed intelligence is mirrored in emerging architectures for space data centers — orbital nodes designed to store, process, and relay data. One concept discussed in recent KP Labs publications is a distributed network of "Space Data Centers" (SDCs) that act like edge computing nodes in orbit. These SDCs could share computing workloads and context, dynamically route data to the satellite with the best ground connection, and allow for nearly continuous availability of insights. In a wildfire monitoring scenario, such a network could reduce response times from hours to minutes and dramatically cut costs by minimizing the need to transmit irrelevant or redundant data. These concepts also include dynamic load balancing, federated learning, and sample classification distributed across multiple spacecraft — further reducing memory and energy burdens on individual units while improving robustness and responsiveness across a mission [1, 2].
But it's important to emphasize that the core idea behind on-board data processing is not merely technical. It's strategic. It allows us to rethink the architecture of space missions, optimize for latency, and design with adaptability in mind. It represents a philosophical shift from centralized control to distributed intelligence. And it opens new avenues for future missions around Earth and the solar system — from autonomous lunar rovers and relay-equipped landers to interplanetary networks that analyze and learn as they go.
At KP Labs, we believe that tomorrow's most impactful space technologies will be smarter, not faster or smaller. By embracing on-board data processing today, we're laying the groundwork for a new generation of more efficient and fundamentally more capable missions. In space, the ability to think — and act — locally will define the next frontier.
Co-authored by: Jakub Nalepa , Marcin Ćwięk (KP Labs)
References
- Gumiela, Michał; Musiał, Alicja; Wijata, Agata M.; Lazaj, Dawid; Weiss, Jonas; Sagmeister, Patricia; Morf, Thomas; Schmatz, Martin; Nalepa, Jakub. (2023). Benchmarking Space-Based Data Center Architectures. In Proceedings of IGARSS 2023 - IEEE International Geoscience and Remote Sensing Symposium. https://ieeexplore.ieee.org/document/10281701
- Wijata, Agata M.; Musiał, Alicja; Lazaj, Dawid; Gumiela, Michał; Przeliorz, Mateusz; Weiss, Jonas; Sagmeister, Patricia; Morf, Thomas; Schmatz, Martin; Longépé, Nicolas; Mathieu, Pierre-Philippe; Nalepa, Jakub. (2024). Designing (Not Only) Lunar Space Data Centers. In Proceedings of IGARSS 2024 - IEEE International Geoscience and Remote Sensing Symposium. https://ieeexplore.ieee.org/document/10642886
- Wijata, Agata M.; Foulon, Michel-François; Bobichon, Yves; Vitulli, Raffaele; Celesti, Marco; Camarero, Roberto; Di Cosimo, Gianluigi; Gascon, Ferran; Longépé, Nicolas; Nieke, Jens; Gumiela, Michał; Nalepa, Jakub. (2023). Taking Artificial Intelligence Into Space Through Objective Selection of Hyperspectral Earth Observation Applications. IEEE Geoscience and Remote Sensing Magazine, 11(2), 70–86. https://ieeexplore.ieee.org/document/10180082
- Ziaja, Maciej; Bosowski, Piotr; Myller, Michał; Gajoch, Grzegorz; Gumiela, Michał; Protich, Jennifer; Borda, Katherine; Jayaraman, Dhivya; Dividino, Renata; Nalepa, Jakub. (2021). Benchmarking Deep Learning for On-Board Space Applications. Remote Sensing, 13(19), 3981. https://www.mdpi.com/2072-4292/13/19/3981
- Nalepa, Jakub; Tulczyjew, Łukasz; Le Saux, Bertrand; Longépé, Nicolas; Ruszczak, Bogdan; Wijata, Agata M.; Smykała, Krzysztof; Myller, Michał; Kawulok, Michał; Kuzu, Ridvan Salih; Albrecht, Frauke; Arnold, Caroline; Alasawedah, Mohammad; Angeli, Suzanne; Nobileau, Delphine; Ballabeni, Achille; Lotti, Alessandro; Locarini, Alfredo; Modenini, Dario; Tortora, Paolo; Gumiela, Michał. (2024). Estimating Soil Parameters From Hyperspectral Images: A Benchmark Dataset and the Outcome of the HYPERVIEW Challenge. IEEE Geoscience and Remote Sensing Magazine, 12(3), 35–63. https://ieeexplore.ieee.org/document/10526314
- Grabowski, Bartosz; Ziaja, Maciej; Kawulok, Michał; Bosowski, Piotr; Longépé, Nicolas; Le Saux, Bertrand; Nalepa, Jakub. (2024). Squeezing Adaptive Deep Learning Methods with Knowledge Distillation for On-Board Cloud Detection. Engineering Applications of Artificial Intelligence, 132, 107835. https://www.sciencedirect.com/science/article/abs/pii/S0952197623020195
More news
Stay informed with our latest blog posts.